RECOMB News: Sentieon Wins PrecisionFDA Multi-omics Data Challenge
The International Conference on Computational Biology (RECOMB) was held at George Washington University from May 4 to 8, 2019, and is one of the most important international conferences in computational biology. During this year’s RECOMB Dream Satellite Conference, the US FDA and NCI announced the results of the PrecisionFDA NCI-CPTAC Multi-omics Enabled Sample Mislabeling Correction Challenge, and awarded the winning team. The challenge had two subchallenges: Subchallenge1 and Subchallenge2. In the first subchallenge, 148 methods were submitted by 51 participants from around the world, and Sentieon won first place. In the second subchallenge, 82 methods were submitted by 30 participants from around the world, and Sentieon tied with two other participants for the highest score. Sentieon was invited to give a keynote speech at the RECOMB Dream Satellite Conference, and more information about the conference can be found at https://dream.recomb2019.org/talks/.
PrecisionFDA Challenge and Sentieon
The US Food and Drug Administration (FDA) has organized several precision medicine data analysis challenges related to genomics on its genomics informatics community and shared data platform, precisionFDA, since 2016. The challenges invite experts from academia and industry worldwide to participate and submit their algorithms. Through these challenges, and post-competition sharing and discussion of methodologies, researchers in the field can explore different multi-omics data analysis methods, provide methodological support for precision medicine, promote innovation, and establish necessary standards.
Sentieon won the precisionFDA Truth Challenge and Consistency Challenge in 2016, the precisionFDA Hidden Treasures – Warm Up Challenge in 2017, and once again won first place in the PrecisionFDA NCI-CPTAC Multi-omics Enabled Sample Mislabeling Correction Challenge organized by precisionFDA in late 2018. Unlike previous challenges, this competition broke through the analysis of a single data type and required participants to analyze the transcriptome, proteome, and clinical phenotype of the same patient from a multi-omics perspective, aiming to establish a model that reflects the relationship between the three types of data within a limited training set using machine learning algorithms.
The Importance of Multi-omics Data Analysis
With the rapid development of omics detection tools, including NGS, acquiring multi-omics big data such as genomics, transcriptomics, proteomics, epigenomics, and phenomics data from the same individual has gradually become a reality. The value of multi-omics data lies in its ability to accurately extract complete information from the data and refine it into a model that can reflect complex molecular interactions. Such models can greatly facilitate researchers’ understanding of diseases, which can then be applied in clinical practice, such as predicting clinical prognosis of tumors through patient genomic and proteomic data.
Currently, the joint analysis of multi-omics is still in its early stages, and its ability to mine weak signals is relatively weak. Machine learning, as a powerful tool for complex data analysis, is gradually being applied. However, how to improve the accuracy of machine learning models in the face of limited training data remains an important issue. One of the main purposes of this challenge was to let numerous participants explore what kind of modeling methods could provide the most accurate results.
Like previous precisionFDA challenges, this competition attracted wide attention from academia and industry, and participating institutions that submitted models included Stanford University, University of Oxford, and more.
The performance and methods of the championship team.
The goal of this challenge is to identify artificially mislabeled samples in the test set based on multiple sets of learning data. Specifically, it is divided into two stages: in the first stage, the protein and clinical phenotype data of the training set and test set are provided, and participants are required to identify the incorrect pairings in the test set. In the second stage, RNA-seq data is added, and participants are required not only to identify incorrect pairings in the test set, but also to correct them.
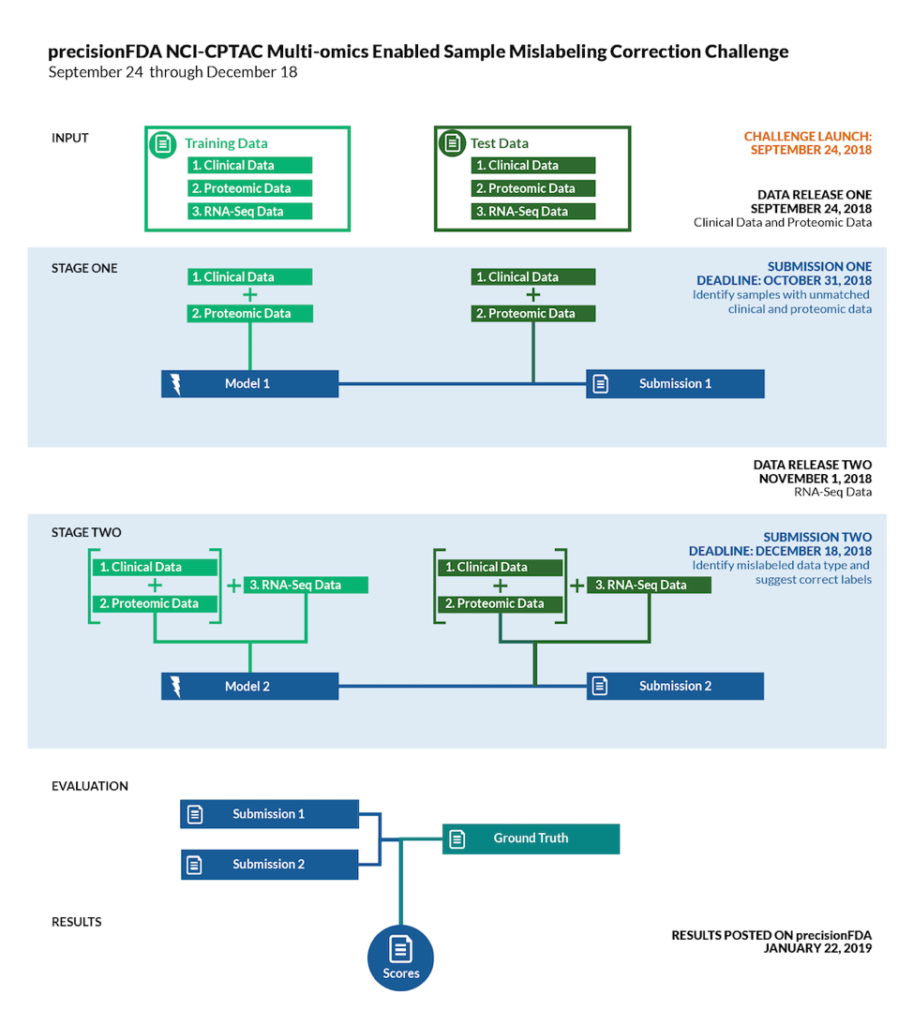
Compare the results of the two submissions with the true set and announce the top three before the challenge website is released:
Subchallenge 1 results: https://precision.fda.gov/challenges/4/view/results

Subchallenge 2 results https://precision.fda.gov/challenges/5/view/results

Renke Pan from Sentieon company won the championship in the first stage and tied for full marks with two other teams in the second stage. Sentieon was also the only participant who entered the top three in both stages.
On May 4th, Sentieon was invited by the challenge organizers to present the model method used at the RECOMB 2019 conference. The abstract has been published on the conference website. (https://dream.recomb2019.org/talks/)
“In Subchallenge 1, for mislabeled sample detection, we apply an ensemble approach, combining statistical inference models such as Least Absolute Shrinkage and Selection Operator (LASSO), Nearest Shrunken Centroid (NSC), and k-Nearest Neighbors (k-NN), to identify mismatched clinical and protein profiles. In Subchallenge 2, for sample mislabeling correction, we use the same machine learning ensemble methodology to give accurate predictions of clinical labels based on the samples’ protein and RNA abundance. In addition, to jointly analyze measurements from both mass spectrometry and RNA-Seq, we build regression models for each gene as a bridge to map the two data types to each other, which enable a novel definition of distance matrix between proteomic and transcriptomic profiling data. The sample mislabeling correction problem is thus reformulated into an optimization problem of matching proteomic and transcriptomic data with the shortest distance. This method achieves perfect correction, assuring “right data for right patient”.
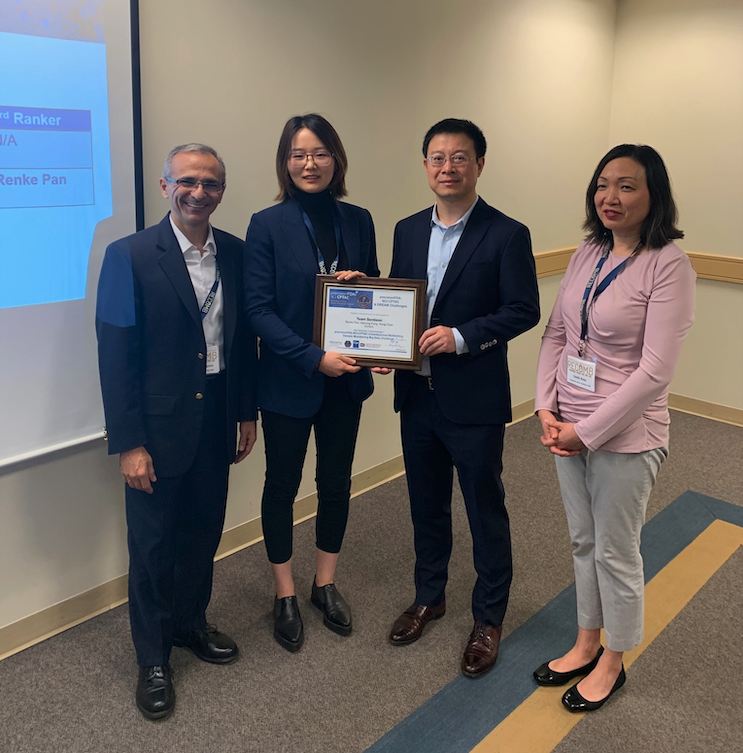
Photo of Renke Pan (second from left) receiving the award on behalf of Sentieon. Left one: Dr. Henry Rodriguez – Director, NCI Office of Cancer Clinical Proteomics Research; Right two: Dr. Bing Zhang – Professor, Baylor College of Medicine; Right one: Dr. Emily Boja – Program Director, NCI Office of Cancer Clinical Proteomics Research.
The application prospects of Sentieon’s machine learning model
In the process of analyzing the challenge data, the Sentieon team developed and used multiple machine learning models to accurately extract weak but definite correlations between different omics data in the background noise.
As we all know, compared with the rapid progress of AI in the field of biological image recognition and non-biological data, the progress in the processing and analysis of omics data, especially genomic data, has always been limited by the number of available truth sets. Currently, there are only less than 10 recognized germline variation truth sets. Somatic variation truth sets need to be maintained by various institutions through dilution and manual review, so the construction cost is high and the data volume is very limited, and the training set of clinical data requires long-term tracking and maintenance.
The excellent AI algorithm development and practice capabilities demonstrated by Sentieon in this challenge, and the ability to model high-dimensional data on a very small sample set, are particularly important in the establishment of biomedical multi-omics models.
Currently, this excellent machine learning algorithm is being applied in Sentieon’s secondary analysis software DNAscope (for germline variation) and TNscope (for somatic variation). On the one hand, this analysis method maintains the stability and accuracy of the DNAseq and TNseq basic algorithms, and on the other hand, it can establish specific models for different sample types, reagent kits, and sequencing platforms based on the sample data provided by the user, greatly improving the accuracy and reliability of specific production environments. We are very willing to collaborate with our partners to explore the application of Sentieon’s omics machine learning algorithms in various medical products.